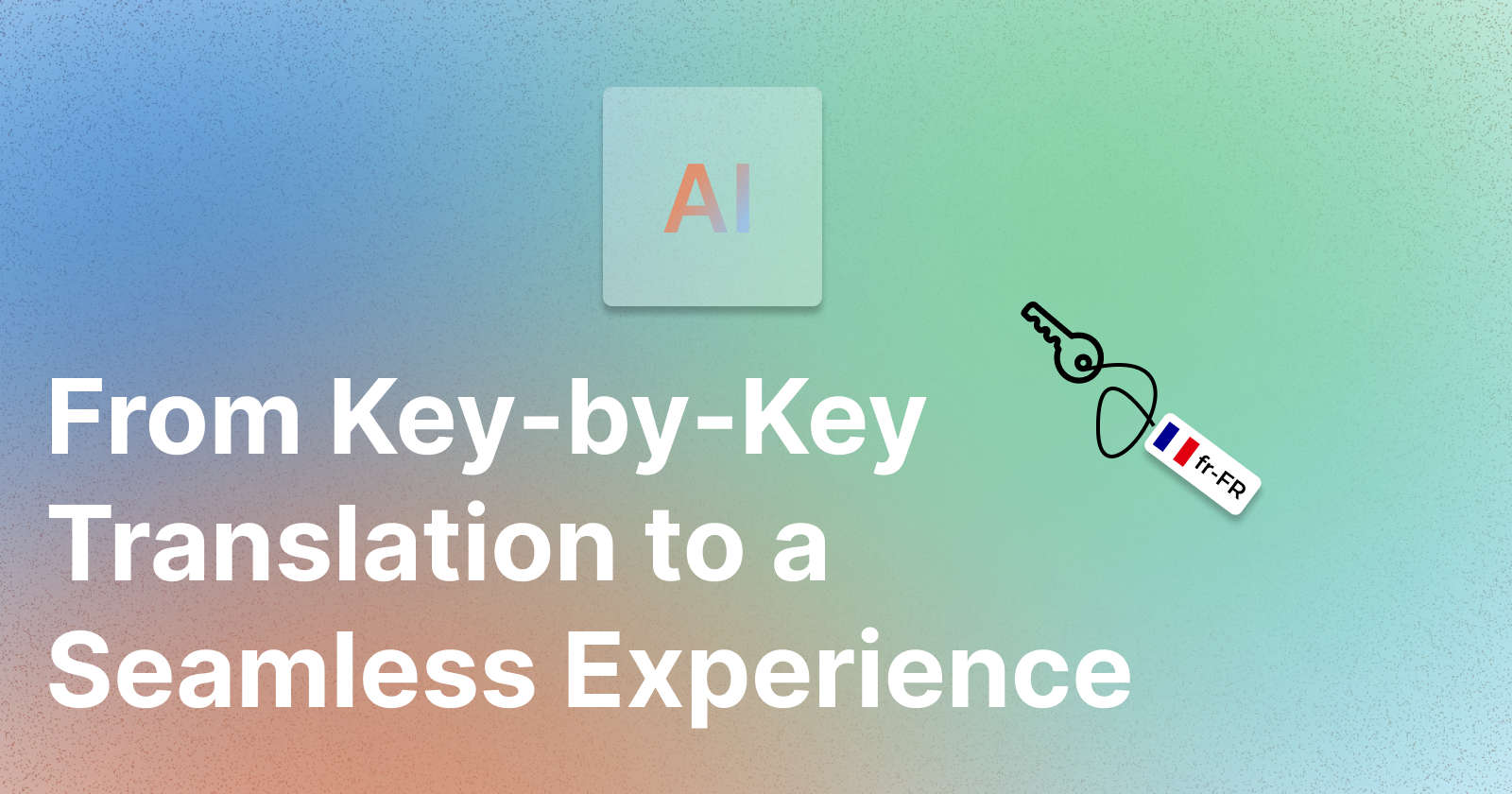
The Limits of Current Translation Solutions
The Difference Between the Two Approaches
Most classic solutions, like DeepL or Google Translate, work on a key-by-key basis (text-element by text-element), where each segment is translated in isolation without considering the overall context of the text. This can lead to inconsistencies in the translation and errors that affect not only the quality but also the integrity of the message.
We wanted to take a more complete approach with contextualized translation, where each word, each sentence, is translated while taking into account the global context. This ensures that the tone, message, and even the subtleties of your content remain consistent and relevant, no matter the target language. We want to show you with examples how this difference is concretely reflected between a key-by-key translation and a more contextualized translation. If you want to learn more about the context before translation, we’ve worked on localization keys and invite you to read this article to explore the topic! Keys, Translation, and Context: Dive Into the Heart of Our Plugin
UX Translation: The Limits of Manual Glossaries
Classic translation solutions often involve complex manual work: creating glossaries, adjusting specific terms for each project, and managing translation keys in a tedious manner.
This approach can really become a nightmare for teams. Imagine having to juggle separate files, inconsistent terms, and constant manual updates. It’s not only time-consuming but also harms the user experience and slows down development teams.
So, we decided to centralize everything to make it smooth. No manual glossary to create, no poorly managed keys. New translations are adjusted thanks to a living translation memory, all directly integrated into your workflow. The goal here is to help you provide a flawless user experience and a well-localized product.
It might sounds a bit weird a ‘living translation memory’, but when you get it, it makes all the difference. Instead of a classic translation memory, that mechanically reuses past translations it’s a much more clever system. With traditional translation memory, even if two sentences have similar meanings, the system won't reuse the translation unless they match exactly. Our live memory recognizes semantically similar content and translates it consistently.
Comparison: DeepL and Google Translate vs. Our Solution
If we take a look at a concrete example, we’ve tested the DeepL Figma’s plugin (basically just a plugin that uses DeepL API) to manage translations directly on Figma. The idea is appealing: automate interface translation without manual copy-pasting. But in practice, limitations persist.
Why? Because the plugin sends each text area individually to the DeepL API. This means that each sentence is translated independently of the others, without any global consistency.
It’s not just about having the right translation (here, one of the ‘remote’ should be translated as a remote TV controller, not remote working), it’s also about keeping global consistency: remote working should be translated the same way everywhere in your product, for instance : ‘Télétravail’.
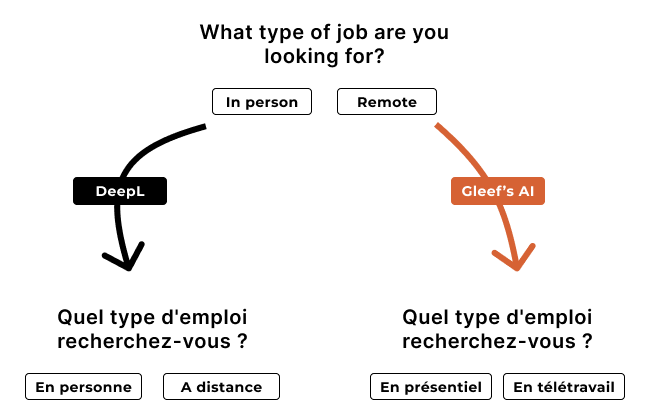

It is clear that an improvement is possible: a system that learns from the choices made and ensures consistency throughout the entire design. To date, no solution fully addresses this need, except Gleef. For more comparisons like this one regarding our latest product, I invite you to read this article which will allow you to concretely compare plugins with each other Why Traditional Localization Tools Are Failing?
I’ve also been asked a lot about Figma’s AI features, especially translation features. Well, it’s going to be amazing, for sure - I didn’t manage to get Beta access yet, so sad - but it won’t solve localization. There will be no translation memory, glossary and other crucial features to keep consistency and reduce human work. It will definitely be awesome for people just looking for a quick and clean translation on a Frame, not for the one looking for having the right word and consistent copy.
A Radical Approach: Bulk Pre-Translating
Why we don’t talk about “pre-translation”, but about final translation for most businesses.
Classic pre-translated solutions rely on an initial step of automatic translation, followed by manual revision. This process can take a lot of time, especially for projects with large volumes. It can also lead to inconsistencies and contextual errors.
With our approach, there is no "pre-translation" in the traditional sense. Our solution generates a final, high-quality, ready-to-use translation, taking into account the global context of the text. This means that the first version is already the right one, without having to go through manual corrections or exhaustive proofreading.
What makes the difference
The quality/cost ratio: Our approach changes the game with a ratio 10x higher than traditional methods. In simple terms, for equivalent quality, our cost is 10 times lower. But most importantly, at the same price, our translation is 10 times better.
Why? Because we take into account the global context from the start. No more manual corrections in loops, fewer back-and-forths, and a much smoother execution.
Human proofreading 10x faster: For those who want human proofreading, it’s simple: our base translation is already super clean, with context well integrated. As a result, corrections become almost instantaneous, and we achieve a 10x time saving overall.
We know it can still be optimized, but we’re making progress. If you have ideas or feedback, we’re all ears.
Our Impact
Why our approach changes the game
What sets us apart from classic localization software is that AI is not just an add-on or a layer. It is truly at the heart of our process, allowing us to create much smarter and more tailored solutions. Moreover, we deeply verticalize our services, which allows us to bring a much richer context at every stage of the process. The result: fewer frictions and more fluidity throughout the entire user experience.
Future Perspectives and Especially Improvement
But we’re not stopping there. AI is a constantly evolving field, and we will continue to improve our promptings and translation methods to go even faster, while boosting quality. Our long-term goal is to minimize friction between the various stakeholders in the process, especially by optimizing marketing touchpoints and maximizing conversion.
We also have a very clear vision: remove the latent friction that often exists around translation keys. We’re already working on it, and we are confident that we will be able to simplify this critical step even further.
Conclusion
Our AI-powered approach is just the start. By focusing on context, consistency, and efficiency, we’re transforming the translation process. We’re committed to continuous improvement, and we’re excited to keep pushing forward and building a better solution together.